I blush to think how I bought into the idea of ‘the singularity’ or ‘superintelligence’ circa 2018. Gloom-and-doom stories expounded from high-status people like Bill Gates, Elon Musk, Stephen Hawking, Ray Kurzweil, Nick Bostrom, and an online community of people who brand themselves rationalists – like Robin Hanson and Scott Alexander – goaded me into thinking it might well be a matter of time before computers take over. Alongside whole institutes – like The Future of Life at MIT and Future of Humanity at Oxford – that are dedicated to ‘AI risk’ and bankrolled by big-tech philanthropists; computer-takeover is an easy assumption to make. Nevertheless, the narrative about intelligence explosions and possible risks obfuscates actual workaday dangers from already existing AI—such as killer robots and misoptimised social media algorithms—that no one has solved and are more important than artificial general intelligence (AGI).
Granted, there are genuine reasons to worry about AGI and one cannot rule out the chances that artificial intelligence takes over just as one cannot rule out the chances that a colossal comet will hit earth or aliens will visit this blue-marvel to see the sights, in this century. But I think we need to gain a sense of proportion about what we concern ourselves with. With the alien and comet example, it seems reasonable to prioritise comet impacts (that actually happen) above alien first contact (that has never happened, but might yet). Especially given that there are more knowns and solutions to addressing a comet-threat than addressing never-seen aliens; aliens who may well be amicable and understanding, or beyond our galaxy, anyway.
Nonetheless, the worriers deserve hearing. Well-informed, confident, and persuasive people like Stuart Russell are concerned. A computer scientist at Berkeley who co-wrote the (text)book on artificial intelligence, Artificial Intelligence a Modern Approach, he laments that artificial intelligence has big ethical issues. Such issues include how to align machine objectives with our own, how to discern correct instructions for machines to never go haywire, how to overcome the anomie of a world where workdays— humans getting out of bed and meeting goals—become obsolete. Russell describes the human complacency regarding artificial intelligence as akin to the indifferent gorillas when our particular human branch took-off and we inadvertently left the gorillas’ habitat devastated. A good point. As we never sought to disenfranchise gorillas (today we even strive to save them from bush-meat hunters) but our past ambitions did them harm all the same; such a scenario with out-of-control machines is similar in that machines’ concerns might leave us lower down the priority list, and thereby do us harm inadvertently or indifferently.
Russell claimed in a Munk debate with opponent Melanie Mitchell, a fellow AI luminary, and protege to Douglas Hofstadder, that “the vast majority of AI researchers are pretty confident it will arrive this century”. So I sought out the data and it turns out that 18% believe future artificial intelligence is “extremely bad”. Bostrom and Mueller, the pollsters, use the minority number who believe in the extremely bad threat to justify research into AI risks and elsewhere into ‘existential risk’ — but the question of whether AI will be an existential threat, meaning a threat to humans’ existence, or what risks best count as existential priority, never comes up in the paper itself. Bostrom and Mueller even leave the ‘never’ answers for superintelligence out of their results table.
Put one way, 82% of 170 eminent researchers (out of 549 pleaded to participate) believe that AI is not going to be an “extremely bad” outcome. Sometimes this data is used to say that researchers nonetheless believe human-level intelligence will arrive by 2040. A median 50% who responded actually do avow that time-frame for human-level AI.
The frame of question for predicting superintelligence, meanwhile, is different in the paper than the media makes out: the question says that assuming machines reach human level intelligence, then how long before superintelligence. . . There are problems with that. For one thing, AI might well not reach human level intelligence—from a recombination of prefit learning competencies in narrow tasks, animal, let alone machine, intelligence has yet to come out—and there is no non-agnostic evidence to think super intelligence would emerge. As Mike Loukides and Ben Lorica phrase it, “A pile of narrow intelligences will never add up to a general intelligence. General intelligence isn’t about the number of abilities, but about the integration between those abilities.” Can anyone point to a non-hypothetical intelligence that has emerged from prior intelligences in a short, intelligently designed, timeframe?
Updated surveys on this topic are coming out this October 2021. But frames for risk again belie an over-focus and echo-chamber reverence for spectacle scenarios with a 105 sample already working in AI safety, rather than on real events, an irony for philosophy and evidence-based inquirers.
Other problems are these:
- The sample contains discrepancy about who gets to make predictions, and define intelligence
- Socio-historical time as-factor is left out
- No random sampled control comparison
3 biologists, 14 cognitive scientists, and 107 computer scientists comprised the ‘expert’ sample, a discrepancy that leaves roboticists and biologists under-represented. The fact that researchers have failed to recreate the intelligence of one c elegans worm with its 307 neurons may put pay to the idea that outcompeting 86bn neurons in the human brain is an impending feat in the embodied breathing world. The idea that intelligent machines being able to beat humans one-on-one may also gain some suspicion when extrapolated to world domination given how many 86bn neuron brains there are in the world, 7.619bn. Being able to win in Go or park a car, for instances, are nice feats, but let us not forget who created Go and cars and algorithms to perform them. Making a Bach concerto from Bach’s materials is still Bach’s material just as grated cheese is still cheesy; as David Cope, the Bachian program’s programmer, nicely puts it. Recombining inputs is not so impressive. The Go pattern maker, autonomous car system, and flashy algorithms, moreover, care about their goals today as much as a watch cares about keeping good time.
It is humans who decide that the watch metaphorically has a ‘goal’ because humans made a goal and reinforced movements that met that goal above alternative movements, like breaking apart, that do not meet the time-keeping ‘goal’; a ‘goal’ for a watch is nonexistent as it just amounts to moving in a set pattern. Yet people have yet to doom-monger over watches’ impressive ability to estimate time better than humans. Likewise a digital thermometer is able to estimate temperature better than humans, but no one doom-mongers that the thermometer’s descendents will decide to kill us for their own good, as some worry about board-game computers. Curiously, this comparison, while a bit silly, says more about humans than about AI: presumably because chess is an adversarial competition it becomes (mis)extrapolated to imply conflict or emotional agency, whereas co-operative watches and thermometers, for all their impressive ability, just never provoke human anxieties.
And the second point? Were the poll conducted in 1948, 58, 68, 78, 88, 98 similar predictions would have been different or similar—and would have turned out to be wrong. Indeed, depending on whether AI was in fashion in the decade (or in an AI winter in researchers’ parlance), the answers would have changed wildy. Consider that a cybernetics thought-collective, in 1956, thought they could make great significant progress in generalising intelligence from calculating machines in a summer; a feat they did not achieve in decades. (The first contemporary programming language came out a year later, Fortran, in 1957.) Consider how Alan Turing predicted, in 1951, that “It seems probable that once the machine thinking method had started, it would not take long to outstrip our feeble powers” because “They would be able to converse with each other to sharpen their wits”. The latter point is interesting, by the way, because it shows how little progress has been made in risk-assessment: ‘innovative’ 21st century decision theorists have put forward that conversing problem, as the self-programming or ‘foom’ effect (from comics’ pictorial sound) as a huge AI risk facing us.
Once upon a time I really did think that I could say there was a ninety percent chance of Artificial Intelligence being developed between 2005 and 2025, with the peak in 2018. This statement now seems to me like complete gibberish. — Eliezer Yudkowksy, self-educated intelligence alignment researcher on cognitive biases.
The random sampling flaw – my third point – entails that laypersons’ guesses provide a comparison for how much is random estimation and how much audacious hype: absolute laypersons I would wager think extremely bad risk science fiction and bad risk a-maybe whereas Musk’s followers would wager catastrophe inevitable; that AI researchers walk between these two paths or waver implies agnosticism rather than ‘worse than nukes’ (Musk’s phrase) or research grant money dedicated to thinking-up God-like AI; instead of that money going towards effective causes and proven risks like malaria bednets, mitigating supervolcano eruption, or honing adolescent trauma therapies. These are evidence-based causes whereas superintelligence and AI risk research is evidence-lacking.
Consider the demographic of people most vocal about AI existential threats. From Tegmark-to-Musk these are men high in socio-economic status and without any pressing, real, risks in their own lives. The only thing that could topple them is no longer proletariat workers but proletariat machines; hence the assumption that humans’ goals control the world, so machines have a star-role to ‘take over’ at all.
Dude, where’s my superintelligence?
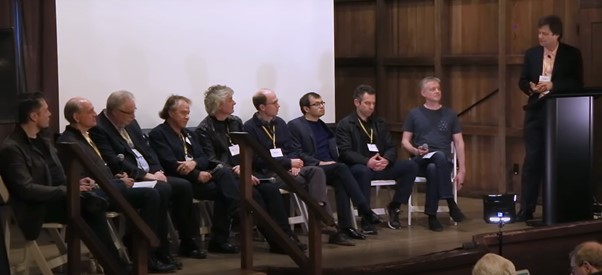
Consider how agents that have-yet-to exist are construed as conquerors, and whose intelligence is cold and calculating, rather than an intelligence boasting emotions and sympathy; in reality, no human-rival intelligence that exists (dolphins, elephants) has intelligence without emotions or co-operative sympathy, so by the reckoning of universal darwinism, it is hard to imagine that even an intelligently designed intelligence would lack convergent emotion or sympathy, or lack intelligent intuition into ethics beyond merely meeting and reweighing ‘goals’. As Russell writes in Human Compatible, consciousness or reflexive awareness is ‘beside the point’ for intelligence. Intelligence he casually defines as goal meeting behaviours.
Arguably, the assumptions of these worriers about what counts as intelligence reveals more about their goals and calculative mentality than the mentalities of these disruptive machines, were they ever to really converge on animal intelligence; prioritising many machine scenario risks above other risks is due to the fantasies of the affluent.
“I say that I don’t worry about AI turning evil is the same reason I don’t worry about overpopulation on Mars […] we’ve never set foot on the planet so how can we productively worry about this problem now?” — Andrew Ng, Machine Learning Pioneer
If you are of low socio-economic status, you cannot foresee the end of the week, let alone the end of the world. There is, then, Identity Politics in Risk Politics. Whereby the most pressing risks and global priorities favour the powerful. Risk reports are written by those least at risk; much as history is written by its victors, so is our present. Thus, artificial intelligence is a risk at the top of lists, such as Oxford’s Future of Humanity Institute and MIT’s Future of Life, whereas inequality or factory farming, for example, are relegated down the cost-benefit triage. Meanwhile the modern day reality of people trafficking and slavery that holds at least 40 million hostage, and risk of a supervolcano blocking out the sun for 72 days, and AI hardware contributing to climate change, are omitted. But these are relegated and omitted by who?
By those who flourish at the top of inequality and those who consume factory farm products and, in the case of AI, by beneficiaries of AI and its risk research grants. But, what is my larger point here?
My larger point is that risk politics is contested rather than the ‘objective morality’ of optimum utility sometimes assumed in influential circles. For example, high profile institutes siphoning funds to the developing world is morally effective but siphoning money to long-shot preventions on the other is ineffective. Such institutes, moreover, never remedy a nation’s internal inequality, and can even parody it. The privileged philanthropist and risk-researcher can make ‘a real difference’ to help those in need through high-impact research and gifted money while carers and childminders forgo sufficient pay to get by in the estate just next door. Granted, keeping an eye-out for high impact long-tail risks is a priority, but how much of the commentary becomes the reality here? Prioritising international strife and ‘AI arms’ races, doom-mongers may reify that which we seek to avoid—as the USA did making missiles like mad for – at the time, imaginary – commentated fear of the USSR building missiles, building them like mad.
Planetary Risks are too often misused as a distraction device from actual real-world problems, especially problems where socio-economic winners are part of the problem rather than campaigners for the solution. Some risks are neglected or dismissed when the planetary risk is less cool or less complex. For example, Nick Bostrom discusses impending intelligence explosions and human enhancement (that arguably, amounts to endorsed eugenics) but forgoes the superficially simple work of campaigning for a ban on killer robots or regulating misused computational statistics or reducing abuse incidents. I do think AI is a threat. But a more mundane, less Faustian, threat for evidence-based reasons:
- Algorithms that corrupt users and steal their time
- Automated inequalities in oppressing poor people and disadvantaged racial groups. Even individuals within groups who work within tech companies
- Self-harm and mental health issues from addictive social-media
- Face recognition that quashes resistance to Big Brother power in Xinjiang
- Technologists abetting states even in the west to prevent citizens having the ability to revolt or rebel if necessary
- A modicum of ability to swing elections in vulnerable states
- Unregulated killing machines autonomously killing people
- Precarious income as jobs don’t disappear but working hours dwindle through automation
- Algorithms used for eugenics ends, such as screening out arbitrary ‘defects’ or designing ‘improvements’
- Widely available 3-D printed guns that are (currently) liable to blow up in the hand after five shots (cold comfort…)
- Unregulated cars and other autonomous entities’ owners not being held adequately responsible for tragic behaviour like car crashes and robots crushing people by accident
These are present-time real-world risks.
Attempting to perform risk research on these has merit. But I also have my doubts that academic research into any one area – like AI governance – has as much power as academics make it out to have: Nature claims that the front-pages of all the hypothetically printed papers piles as high as Mount Kilimanjaro. It takes activism and movements to make changes, sometimes along with co-operative academic work helping causes find compelling justifications for social movements.
The above risks are hard to rank, since, in scale, social media I intuit is worst but potential worse hazards include printed weapons, and killing machines. The real question deserving of research and funding – in terms of an evidence-based catastrophe outcome – is leading a movement to address how member states at the United Nations can come to agree on lethal autonomous weapons (LAWs) bans. Other simpler problems like algorithms, can undergo federal drug administration style scrutiny. And even the long-tail big risks from AI—these do deserve some attention to pre-empting its risks. (But let us please keep it proportional and practical.) I contend that such attention entails affirmative action schemes whereby those without zeal for AI and marginalised demographics comprise the people working on AI and risks; Naomi Oreskes and Helen Longino make a compelling case for standpoint epistemiology serving society and preventing the corruption of any one group coming to dominate in a scientific, and stakes-loaded, endeavour. Secondly, I contend that, as the newest edition of Russell’s textbook, Artificial Intelligence, suggests, all programmers work on the problem to draw up an ethical ‘constitution’ for AI projects whereby human preferences, however difficult to define, are considered as an alignment goal from the start lest things, in a improbable scenario, spin out of control and we must scramble to pull too many (metaphoric) plugs, too late.
Nevertheless, as argued here, there are more pressing and workaday threats than future AI, such as dating app abuse and even catastrophic verifiable risks like supervolcano eruption throwing earth into an ice age – and a global famine. There is enough at stake already in practical risk research. If we cannot yet solve the contemporary alignment problems for the risks listed above: why is it evidence-based, and rational, to risk human-level intelligence—and beyond—at all?
Because ‘we can’ and AI might just be our dream saviour against labour and other mega risks while it might just be our nightmare doom, is hardly a good explanation; at least, when you minus human males’ Muskian megalomania from the cost-benefit equation.